Harnessing the Power of Agriculture Datasets for Machine Learning
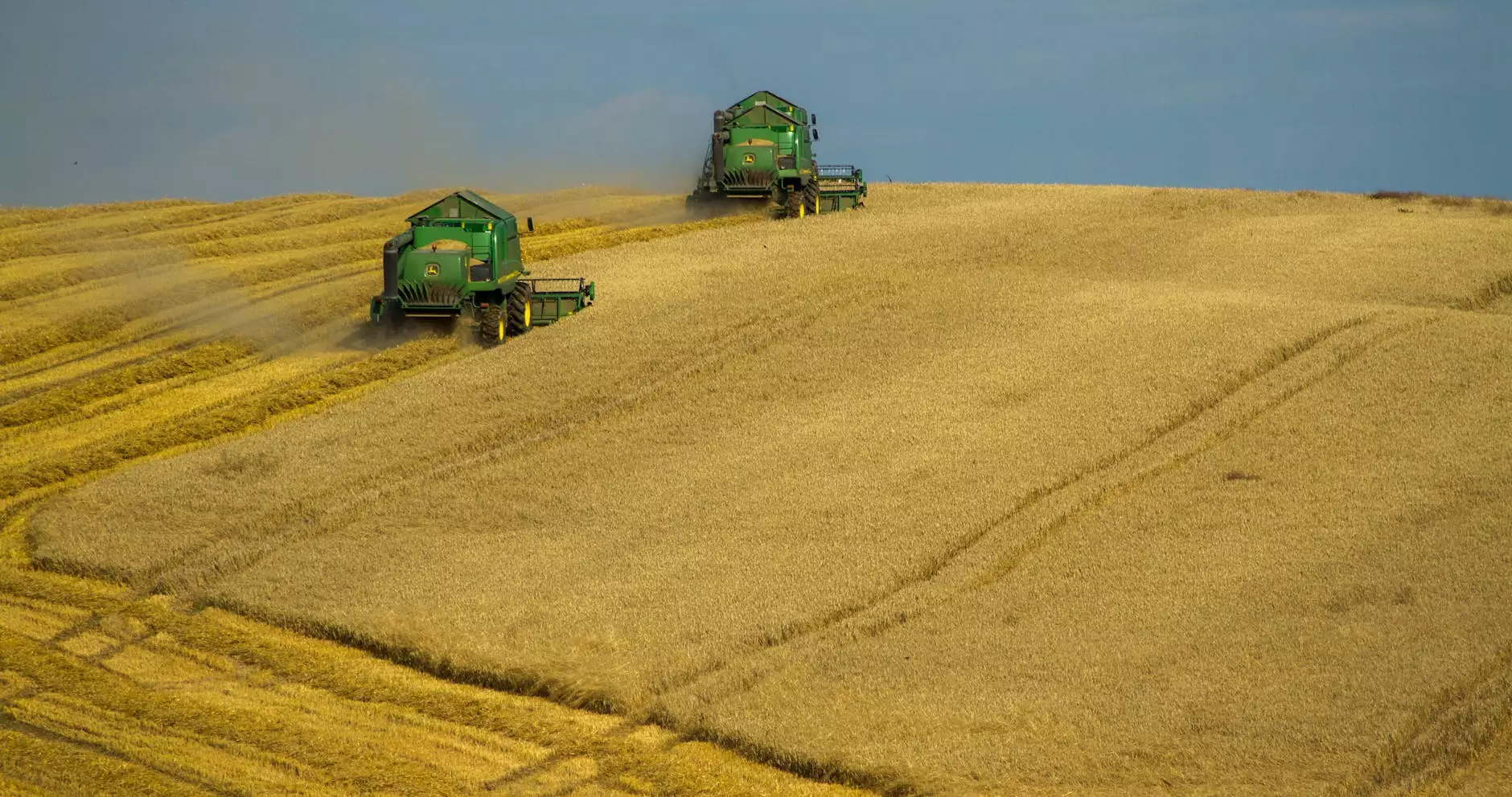
In the rapidly evolving world of technology and business, machine learning is at the forefront, driving innovation across various sectors. One of the most impactful applications of machine learning is in the field of agriculture. The integration of agriculture datasets for machine learning is transforming traditional farming practices and empowering agricultural businesses to optimize their operations, improve yields, and make data-driven decisions.
The Importance of Data in Agriculture
As the global population continues to grow, the demand for food increases, placing immense pressure on the agricultural industry. Data-driven solutions are essential for meeting this demand sustainably. The use of agriculture datasets allows farmers and agricultural businesses to:
- Enhance crop productivity
- Manage resources more efficiently
- Predict weather patterns
- Detect diseases and pests early
- Optimize supply chains
Types of Agriculture Datasets
There are various types of datasets that are valuable for machine learning applications in agriculture. Understanding these can help businesses choose the right data to achieve their goals. Here are some key categories:
1. Climate and Weather Data
Climate data includes temperature, humidity, rainfall patterns, and seasonal variations. This information helps farmers make informed decisions about planting and harvesting times. Access to reliable weather forecasts through data analytics can significantly improve productivity.
2. Soil Data
Soil datasets provide insights into soil health, nutrient levels, and moisture content. Machine learning models can analyze this data to recommend optimal fertilization and irrigation practices, leading to better crop yields.
3. Crop Data
This includes historical data on crop yields, types of crops grown, and performance under various conditions. Using historical crop data, machine learning algorithms can predict future yields and suggest crop rotation strategies that enhance soil health.
4. Satellite and Remote Sensing Data
Remote sensing technology captures data about crops and land use from satellites. This information can be analyzed using machine learning to monitor crop health, assess land degradation, and plan for sustainable land management.
5. Market and Economic Data
Understanding market trends and consumer behavior is crucial for agricultural businesses. By analyzing economic data related to prices, demand, and supply, businesses can make better marketing and sales strategies.
Utilizing Agriculture Datasets in Machine Learning
To effectively implement machine learning in agriculture, organizations must focus on two primary aspects: data acquisition and model development. Here’s how to approach both:
Data Acquisition
Collecting a comprehensive and relevant dataset is critical. Businesses can obtain agricultural datasets from various sources:
- Government Agencies: Many governments provide open-access datasets related to agriculture.
- Research Institutions: Universities and research organizations often conduct studies that result in valuable datasets.
- Commercial Providers: Several companies specialize in collecting and selling agricultural data.
- Data Collaboration: Collaborating with other farmers and businesses can lead to more extensive datasets.
Model Development
Once the data is collected, it's essential to develop appropriate machine learning models. Here’s a general approach:
- Data Preprocessing: Clean the data to remove inaccuracies and fill in missing values.
- Feature Selection: Identify the most relevant features that will enhance model performance.
- Choosing the Right Algorithm: Select suitable machine learning algorithms based on the problem statement (e.g., regression for yield prediction, classification for disease detection).
- Training and Testing: Split the dataset into training and testing sets to validate the model’s performance.
- Model Refinement: Iterate on the model by fine-tuning parameters and incorporating feedback.
Case Studies: Successful Applications of Machine Learning in Agriculture
Real-world examples showcase the incredible potential of utilizing agriculture datasets for machine learning. Here are a few noteworthy applications:
1. Precision Farming
Precision farming utilizes data analytics and machine learning algorithms to enhance crop productivity. For instance, a company might use soil data, weather predictions, and yield history to create precise irrigation schedules, ultimately saving water and increasing yields.
2. Pest and Disease Prediction
Machine learning models can analyze environmental conditions and historical data on crop diseases to predict outbreaks. This allows farmers to take preventative measures before an outbreak occurs, protecting their crops and reducing pesticide use.
3. Crop Yield Prediction
Farmers can use machine learning to predict the yield of different crops based on factors such as weather, soil conditions, and historical yield data. This ability to forecast yields can help with planning and resource allocation, minimizing waste and improving profitability.
Challenges and Solutions in Data Utilization
Despite the myriad benefits of using agriculture datasets for machine learning, several challenges remain:
Data Quality and Availability
One of the most significant challenges is ensuring the quality and availability of data. Inaccurate data can lead to misleading outcomes. To combat this, businesses should focus on:
- Standardizing data collection methodologies
- Collaborating with trusted data providers
- Regularly updating datasets to reflect current conditions
Understanding Machine Learning
Many agriculturalists may not have a strong background in data science and machine learning. Bridging this knowledge gap is crucial:
- Providing training programs for farmers
- Partnering with data scientists to develop user-friendly solutions
- Engaging in community-based projects to raise awareness
The Future of Agriculture and Machine Learning
The integration of agriculture datasets for machine learning represents a significant leap towards a more sustainable agricultural future. As technology advances, we will likely see more sophisticated applications, including the use of:
- Artificial Intelligence: AI algorithms can further enhance decision-making processes by analyzing large datasets in real-time.
- IoT Devices: Internet of Things (IoT) devices can collect data directly from the field, providing real-time insights into soil and crop conditions.
- Blockchain: Leveraging blockchain technology can ensure data integrity and traceability, building trust among all stakeholders in the supply chain.
Conclusion
In conclusion, the use of agriculture datasets for machine learning offers a wealth of opportunities for businesses in the agricultural sector. By harnessing the power of data, companies can drive innovation, increase efficiency, and contribute to sustainable farming practices that meet the challenges of a growing global population. As we look to the future, the fusion of technology and agriculture will undoubtedly reshape the landscape of food production, offering sustainable solutions for generations to come.
Businesses like Keymakr are at the forefront of this transformation, combining software development expertise with industry-specific datasets to empower agricultural businesses with the tools they need to succeed in an increasingly data-driven world. The journey into the future of agriculture is just beginning, and those who embrace these changes will be the leaders of tomorrow.
agriculture dataset for machine learning