Unlock the Power of Data with Advanced Annotation Tools and Platforms
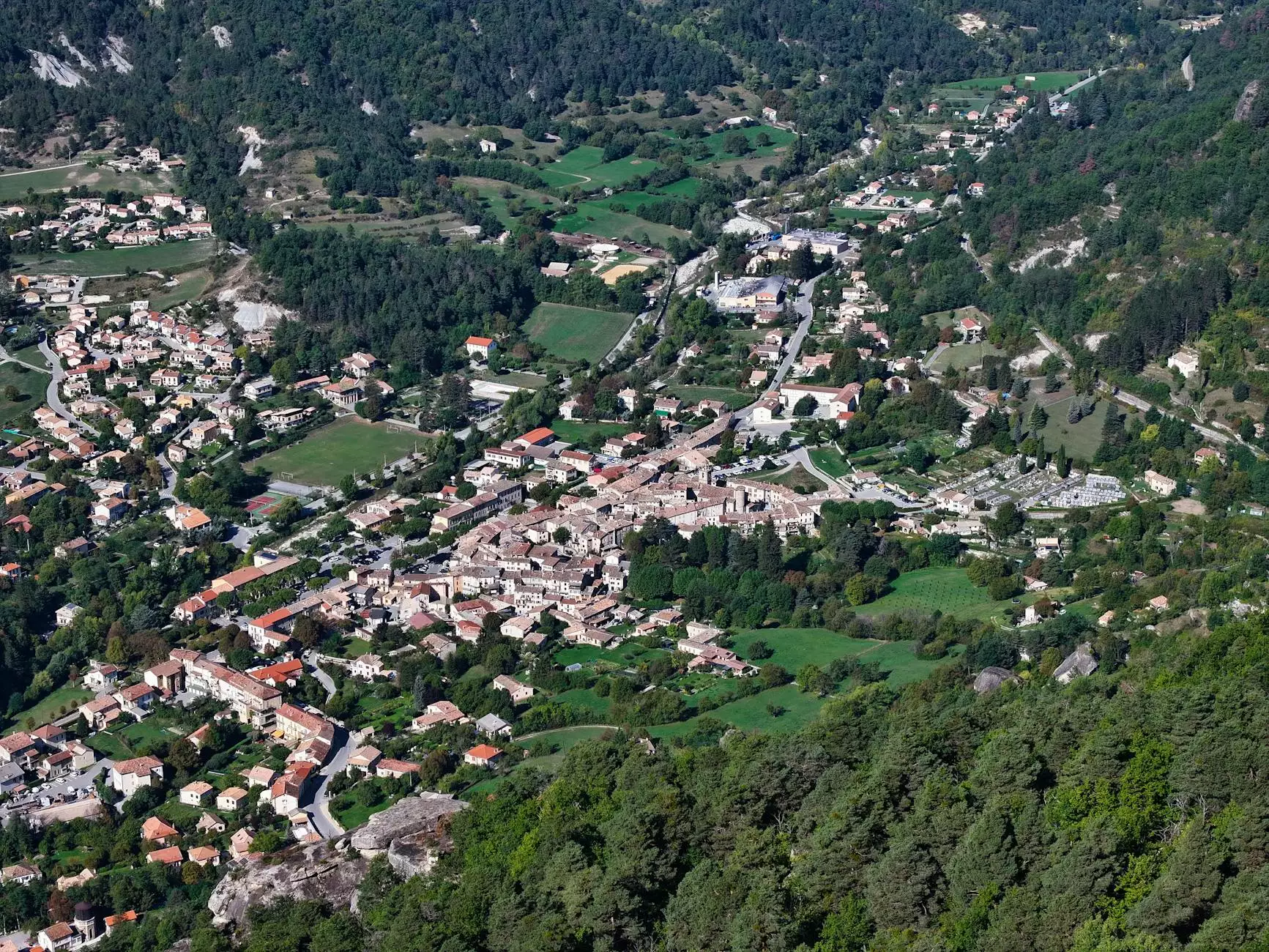
Data is the backbone of machine learning and artificial intelligence. Properly annotated datasets are crucial for the successful training of models, ensuring that they learn accurately and effectively. The importance of data annotation tools and platforms cannot be overstated. These tools streamline the annotation process, enhancing accuracy and efficiency, allowing businesses to focus on what truly matters: obtaining insights and making informed decisions.
What is Data Annotation?
Data annotation is the process of labeling data for use in machine learning algorithms. This can involve various forms of data, including images, text, and audio files. Properly annotated data allows algorithms to learn from patterns and make predictions based on new, unseen data.
The Importance of Data Annotation
In today's data-driven world, effective data annotation ensures that AI models are robust and reliable. Let's explore a few reasons why this process is vital:
- Enhanced Model Performance: Accurate annotations lead to better model accuracy and reliability.
- Improved Training Efficiency: High-quality data reduces the training time of machine learning models.
- Scalability: Automated data annotation tools allow teams to scale their efforts, handling large volumes of data without sacrificing quality.
Exploring Data Annotation Tools
There are countless data annotation tools available today, each offering unique features to meet different needs. At keylabs.ai, we understand that choosing the right tool can significantly impact your project’s success. Here are some top features to look for:
User-Friendly Interface
Choosing a data annotation tool with a user-friendly interface can drastically reduce the learning curve for your team. The easier it is to navigate and use the tool, the faster your team can get to work.
Customization Options
Every project has different requirements. Look for tools that offer customization options to tailor the annotation process according to your specific needs. This can include custom labeling schemes, workflows, and annotation formats.
Integration Capabilities
Your data annotation tool should seamlessly integrate with other software and platforms your team uses. This improves productivity by ensuring a smooth flow of data between different systems.
Quality Assurance Features
To maintain high standards, quality assurance features are essential. Tools that include options for reviewing and validating annotations help ensure the accuracy and reliability of your datasets.
Types of Data Annotation Platforms
Data annotation platforms can be categorized based on the type of data they handle. Here are some common types:
Image Annotation Platforms
These platforms focus on annotating images for tasks such as object detection, image segmentation, and image classification. The goal is to provide clear labels that AI models can learn from.
Text Annotation Platforms
Text annotation tools are essential for handling natural language processing tasks. They can annotate data for sentiment analysis, entity recognition, and more, allowing AI to understand context and meaning.
Audio Annotation Platforms
For speech recognition and audio analysis, audio annotation platforms provide the ability to label audio files accurately, which is crucial for natural language understanding tasks.
Choosing the Right Data Annotation Tool
When selecting a data annotation tool, consider the following:
- Your Team's Needs: Assess what features your team will benefit from most.
- Budget: Analyze the cost against your budget and the value the tool provides.
- Scalability: Ensure the tool can grow with your data annotation needs.
- Customer Support: Opt for platforms that provide excellent customer service and support.
Benefits of Using a Data Annotation Platform
Investing in a robust data annotation platform can deliver numerous benefits:
- Increased Accuracy: Tools designed specifically for annotation reduce human error.
- Time Efficiency: Automation features can greatly speed up the annotation process.
- Cost-Effectiveness: Reduces the need for extensive manual input.
Case Studies: Successful Implementation of Data Annotation
Many businesses have harnessed the power of data annotation tools to achieve their goals. Here are a couple of examples:
Case Study 1: Retail Industry
A leading retail company used a data annotation platform to improve its product recommendation system. By annotating user behavior data, they were able to personalize experiences that increased customer satisfaction and boosted sales.
Case Study 2: Autonomous Vehicles
An automotive company employed an image annotation tool to label thousands of images collected from its fleet of autonomous vehicles. This labeled data was crucial for training their machine learning models, enabling significant advancements in safety features.
Future Trends in Data Annotation
The future of data annotation is bright and full of potential. Here are some trends to watch:
- AI-Assisted Annotation: AI will play a more significant role in automating the annotation process, significantly reducing manual effort.
- Increased Demand: As AI continues to grow, so will the demand for quality annotated data across various industries.
- Focus on Privacy and Ethics: With data privacy becoming more critical, there will be an emphasis on ethical data annotation practices.
Conclusion
In conclusion, data annotation is a critical component in the machine learning pipeline. Investing in the right data annotation tool or platform can dramatically impact the success of your AI projects. At keylabs.ai, we are committed to providing cutting-edge solutions that meet the diverse needs of businesses looking to leverage data for superior outcomes. Implementing robust data annotation processes is not just about keeping up; it's about leading the charge into the future of technology.
Embrace the power of data annotation and watch your business transform today!
https://keylabs.ai/