Enhancing Security with Malware Detection Machine Learning at Spambrella
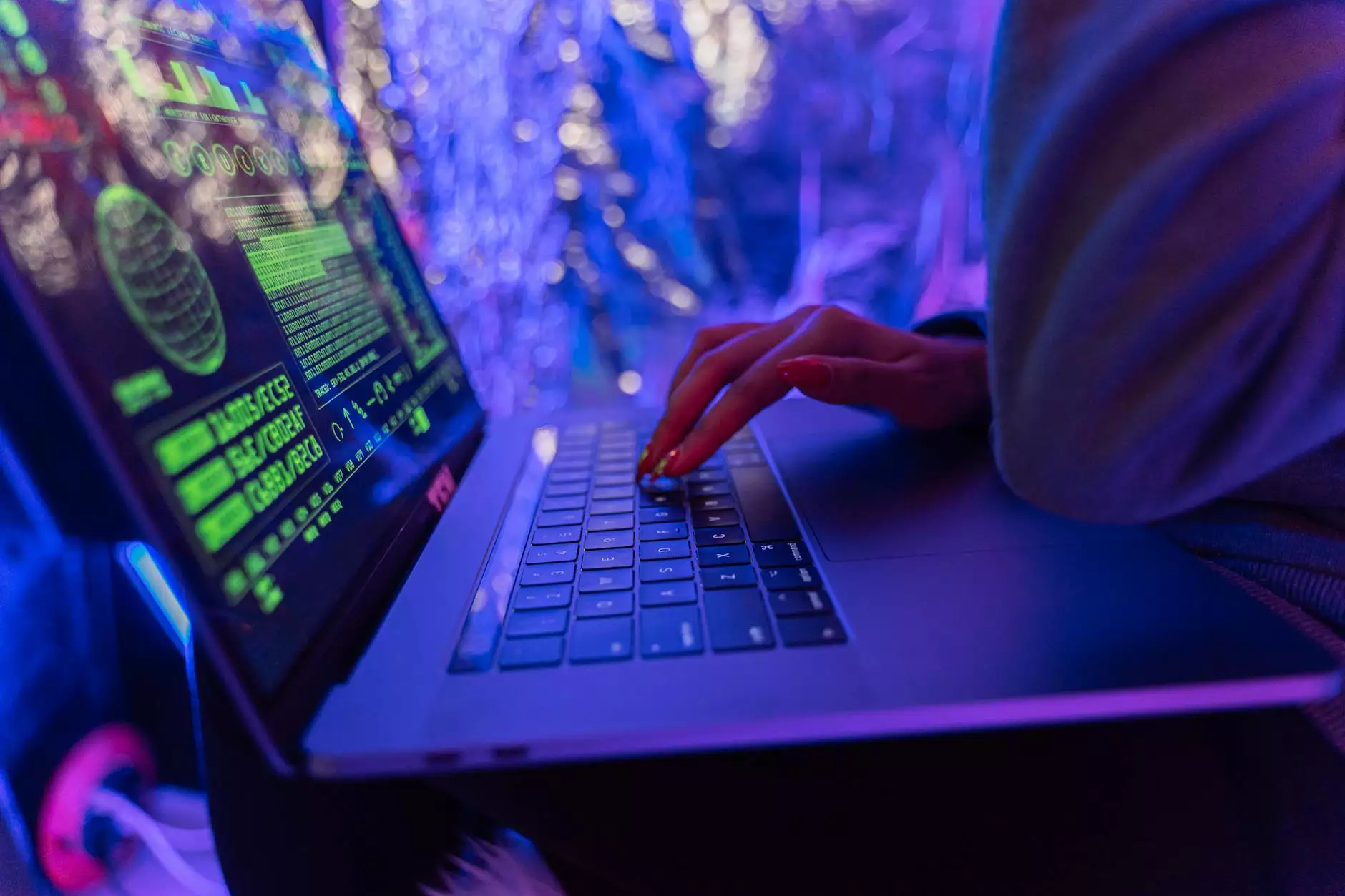
In today's digital age, cybersecurity is of paramount importance for both businesses and individuals. As cyber threats evolve, so too must our methods of detection and prevention. One of the most promising advancements in this field is the use of malware detection machine learning. In this article, we will delve into how Spambrella leverages this technology to provide cutting-edge IT services and security solutions, keeping your data safe and secure.
Understanding Malware and Its Impact on Businesses
Malware refers to any software intentionally designed to cause damage to a computer, server, client, or computer network. This includes various forms such as viruses, worms, Trojans, ransomware, and spyware. The consequences of a malware attack can be devastating, leading to data loss, financial theft, and severe impacts on reputation. Businesses must take proactive measures to safeguard their systems and data from these threats.
Common Types of Malware
- Viruses: Malicious code that attaches itself to clean files and spreads throughout a computer system.
- Worms: Standalone software that replicates itself to spread to other computers.
- Trojans: Disguised as legitimate software but perform harmful actions once activated.
- Ransomware: Locks files and demands payment to unlock them.
- Spyware: Secretly collects user information through the user's internet connection.
Why Traditional Detection Methods Are Insufficient
Traditional malware detection techniques often rely on signature-based detection. This involves creating a database of known malware signatures and frequently updating it. While effective for known threats, this approach falls short when it comes to identifying new or modified forms of malware. Attackers are adept at camouflage, frequently altering their software to evade traditional defenses.
Limitations of Signature-Based Detection
- High False Negative Rates: New malware strains might go undetected due to lack of existing signatures.
- Dependence on Updates: Requires constant updates to remain effective against emerging threats.
- Slow Response Time: Often reacts to threats only after they have already caused damage.
The Role of Machine Learning in Malware Detection
Machine learning (ML) is a subset of artificial intelligence that involves the use of algorithms and statistical models to allow computers to perform specific tasks without explicit instructions. The application of malware detection machine learning represents a significant breakthrough in cybersecurity.
How Machine Learning Works in Malware Detection
- Data Collection: Machine learning models require large datasets containing examples of both benign and malicious software.
- Feature Extraction: Essential characteristics or features of the software are identified and used to train the model.
- Training the Model: The model learns to differentiate between benign and malicious software based on the features extracted.
- Real-Time Analysis: Once trained, the model can analyze software in real-time, identifying potential threats based on learned patterns.
Benefits of Using Machine Learning for Malware Detection
- Increased Accuracy: Machine learning models can analyze vast amounts of data with higher precision, reducing the occurrence of false positives and false negatives.
- Adaptive Learning: These systems continuously learn from new data, enabling them to adapt to evolving threats.
- Behavioral Analysis: Instead of relying solely on known signatures, ML can identify malicious behavior based on the actions of the software.
Spambrella's Approach to Malware Detection
At Spambrella, our commitment to cybersecurity is unwavering. We have integrated malware detection machine learning into our suite of IT services and computer repair solutions, ensuring our clients are protected against both existing and emerging threats.
Our Methodology
- Comprehensive Threat Intelligence: We gather data from various sources, continuously updating our knowledge base on the latest malware trends.
- Cutting-Edge Machine Learning Algorithms: Utilizing advanced algorithms, we can analyze software behavior and detect anomalies indicative of malware.
- Real-Time Monitoring: Our systems operate in real-time, allowing for immediate action against detected threats.
- Client Education: We believe in empowering our clients with knowledge, providing them with insights about the malware landscape and preventive measures.
Case Studies: Real-World Applications
To illustrate the effectiveness of our machine learning approach, let’s look at some case studies highlighting how Spambrella has successfully deployed malware detection machine learning in various contexts.
Case Study 1: Financial Sector Security Enhancement
A regional bank faced frequent phishing attempts and malware attacks that targeted customer data. Spambrella implemented a machine learning-based detection system that analyzed and flagged suspicious activities in real-time. As a result, the bank reported a 70% decrease in successful phishing attacks within the first quarter of deployment.
Case Study 2: Healthcare Data Protection
In a healthcare organization, patient data is critically sensitive. Spambrella's machine learning algorithms monitored data access patterns and identified anomalous behavior, preventing potential breaches before they could escalate. The organization experienced zero data breaches in a year, showcasing the reliability of machine learning in protecting sensitive information.
Future Trends in Malware Detection and Machine Learning
The future of malware detection machine learning looks promising as advancements in technology continue to reshape the cybersecurity landscape. Here are some emerging trends that are likely to shape the field:
1. Increased Use of Deep Learning
Deep learning, a subset of machine learning, utilizes neural networks to analyze data; it's expected to enhance malware detection accuracy and speed further.
2. Integration with Other Technologies
As cyber threats become more sophisticated, integrating machine learning with technologies like big data and the Internet of Things (IoT) will be crucial for holistic security solutions.
3. Focus on Automation
With automation, responses to detected malware can be instantaneous, minimizing potential damage and reducing the need for human intervention.
4. Collaborative Defense Mechanisms
Organizations may adopt collaborative frameworks where threat information is shared across sectors, allowing for more effective defense strategies and faster adaptation to new threats.
The Spambrella Advantage
Choosing Spambrella means opting for a partner that prioritizes your security through innovative technologies. Our malware detection machine learning solutions are designed to keep your systems secure, allowing you to focus on what you do best: running your business.
Why Partner with Us?
- Expertise: Our team consists of seasoned professionals dedicated to cybersecurity.
- Customized Solutions: We tailor our services to fit your unique business needs.
- Proven Results: Our success stories speak volumes about the effectiveness of our solutions.
Conclusion: Secure Your Future with Spambrella
The integration of malware detection machine learning in your cybersecurity strategy is no longer optional; it is essential. As threats grow more sophisticated, employing a proactive, machine learning-based approach ensures that your systems remain resilient.
At Spambrella, we understand the importance of maintaining secure IT environments and are dedicated to providing comprehensive security solutions tailored to your business needs. Partner with us today for a safer tomorrow.